Predictive personalization is a strategy used by e-commerce businesses to tailor the shopping experience for individual customers based on their past behavior and predicted future actions. By analyzing data on customer interactions, such as what products they have viewed or purchased in the past, businesses can make informed guesses about what a customer is likely to be interested in and provide personalized recommendations and marketing messages. The idea of predictive personalization is so commonplace by now because of the pervasiveness of Netflix and Amazon we're almost surprised when an online experience doesn't provide it. Predictive personalization has become a baseline customer expectation in e-commerce both to drive sales and improve the shopping experience.
Some common use cases:
- Amazon uses predictive algorithms to recommend products to customers based on their browsing and purchase history, resulting in a 35% increase in sales.
- Netflix also uses predictive personalization to recommend TV shows and movies to its users, leading to a 75% increase in content engagement.
- Spotify uses predictive personalization to create personalized playlists for its users based on their listening history and preferences, resulting in a higher retention rate and increased revenue.
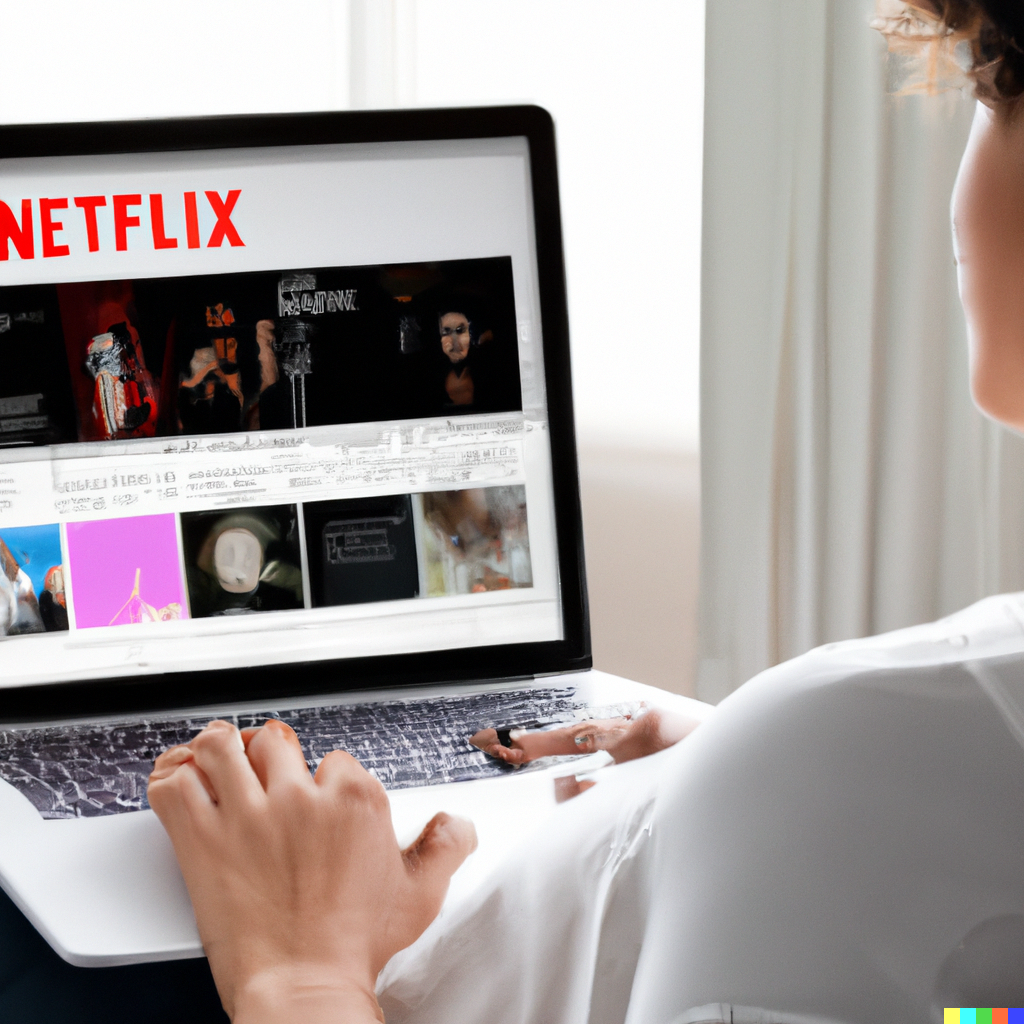
How to use it
There are a few ways in which predictive personalization can be implemented in e-commerce. If there's enough web traffic to make personalization useful, machine learning algorithms can analyze customer data and make predictions about their future behavior. For example, an e-commerce business might use a predictive model to predict what products a customer is likely to purchase based on their past purchases and browsing history. When we've A/B tested email campaigns for clients that implemented predictive models based on past purchase behavior, for instance, we've seen an increased click-through rate (CTR) on our messages which leads to more time spent on the site and increased revenue
Another approach to predictive personalization is to use customer segmentation to group customers into categories based on their characteristics and behavior. This allows businesses to tailor their marketing and recommendations to specific segments of customers, rather than trying to appeal to all customers in the same way. Common segments we use might be retail versus wholesale/trade customers, VIP (high LTV) customers versus customers who only buy on sale or using a discount, or purchasers by product category. This knowledge of customer cohorts can also be useful in prioritizing the limited time and resources of staff so that their time is spent focused on the highest-value customers with a high intent to purchase.
One benefit of using predictive personalization in e-commerce is that it can help businesses to increase sales and customer loyalty. By providing customers with personalized recommendations and marketing messages that are relevant to their interests and needs, businesses can improve the overall shopping experience and increase the likelihood of making a sale.
In addition, predictive personalization can also help businesses to save time and resources by automating certain tasks, such as creating personalized email campaigns or targeting specific customers with ads. This can allow businesses to focus on other areas of their operations, such as product development or customer service.
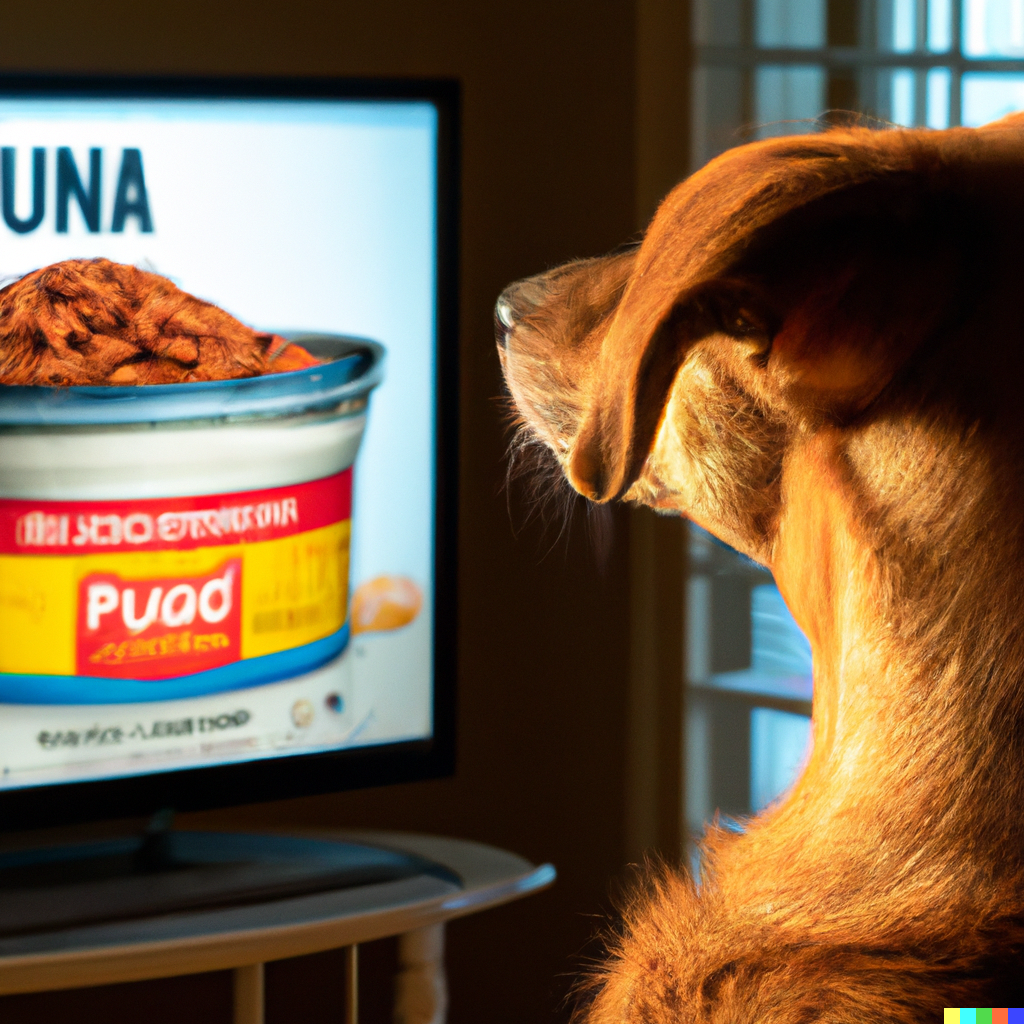
Privacy and Compliance
In order to comply with privacy regulations in specific regions like California or the EU, e-commerce businesses that use predictive personalization must be transparent about how data is collected and used. This includes providing clear and easily accessible privacy policies, as well as obtaining consent from customers before collecting and using their data.
In California, businesses are required to comply with the California Consumer Privacy Act (CCPA), which gives residents of the state certain rights over their personal information, such as the right to know what personal information is being collected about them and the right to request that it be deleted. In the EU, businesses are required to comply with the General Data Protection Regulation (GDPR), which gives EU citizens similar rights over their personal information. Both the CCPA and GDPR also require businesses to report data breaches to affected individuals and regulatory authorities.
To ensure compliance, it is recommended to work with legal counsel to review and update current privacy policies and practices, and to conduct regular audits to ensure that personal data are being handled in accordance with regulations. Additionally, businesses should also consider implementing technical measures to protect personal data, such as encryption and access controls.
Since predictive personalization models are implemented at scale and are generally off-the-shelf software products offered by trusted third parties, there's little likelihood that privacy issues will be a concern. With that said, respecting customer privacy and being transparent about how data is collected and used in accordance with California or EU regulations should always be standard operating practice.
Some common e-commerce predictive personalization off-the-shelf software
Nosto and A/B Tasty are representative of the types of off-the-shelf tools that are used in e-commerce, though they serve different purposes.
Nosto is a predictive personalization platform that uses artificial intelligence and machine learning to analyze customer data and make predictions about customer's behavior. This information is then used to create personalized recommendations and marketing messages that are tailored to individual customers' interests and needs. Some of the features of Nosto include product recommendations, personalized email campaigns, and targeted ads.
AB Tasty, on the other hand, is a tool for A/B testing and optimization. It uses AI to power experimentation & personalization, feature management, and product optimization. A/B Tasty allows e-commerce businesses to test different versions of their website and marketing campaigns to see which ones perform the best. This can include testing different headlines, images, and layouts of a website, as well as different copy, subject lines, and calls-to-action in an email campaign. The goal of A/B testing is to identify the elements of a website or campaign that are most effective in converting visitors into customers.
How it works
The specific algorithms used for predictive personalization can vary, but some common methods include collaborative filtering, content-based filtering, and hybrid methods that combine both techniques.
Collaborative filtering uses the behavior of similar customers to make recommendations, while content-based filtering uses the characteristics of the products themselves. Algorithms like Random Forest, SVM, Neural Network and Gradient Boosting can be used to build the predictive model. These algorithms use historical data and learn patterns from it to make predictions about future behavior. It is important to note that the technical implementation of predictive personalization should also consider privacy regulations and best practices for data collection and use.
What to watch out for
Predictive personalization, while a powerful tool for e-commerce businesses, does have some downsides to consider. Some of these include:
Privacy concerns: Predictive personalization relies on collecting and analyzing data on customer interactions, which can raise concerns about privacy. Businesses must ensure that they are transparent about how data is collected and used and that they comply with relevant privacy regulations such as GDPR or CCPA.
Bias: Predictive personalization models can perpetuate bias if the data used to train them is biased. For example, if the historical data used to train a model is mostly from a certain demographic, the model may be less accurate for other demographics.
Over-personalization: Predictive personalization can result in a "filter bubble" where customers are only exposed to products or content that aligns with their past behavior and interests. This can limit their exposure to new and diverse products or ideas.
Dependence on data: Predictive personalization models require large amounts of data to be accurate, which can be difficult for small e-commerce businesses with limited web traffic. In addition, predictive personalization can become less effective when the data is not updated or refreshed frequently.
Dependence on Algorithm: Predictive personalization models also depend on the algorithm used to build the model. Some algorithms might not be suitable for certain types of data or might be less accurate in certain situations.
Limitation on Creativity: Predictive personalization can also limit the creativity of businesses as they might rely too much on the data and algorithm to make decisions.
Overall, predictive personalization is a powerful tool for e-commerce businesses looking to improve the shopping experience for their customers and drive sales. By using data and machine learning algorithms to make informed predictions about customer behavior, businesses can tailor their marketing and recommendations to individual customers and increase customer loyalty. Far from being a nice-to-have feature of some of the largest websites, customers have come to expect this level of personalization from brands they know and trust.